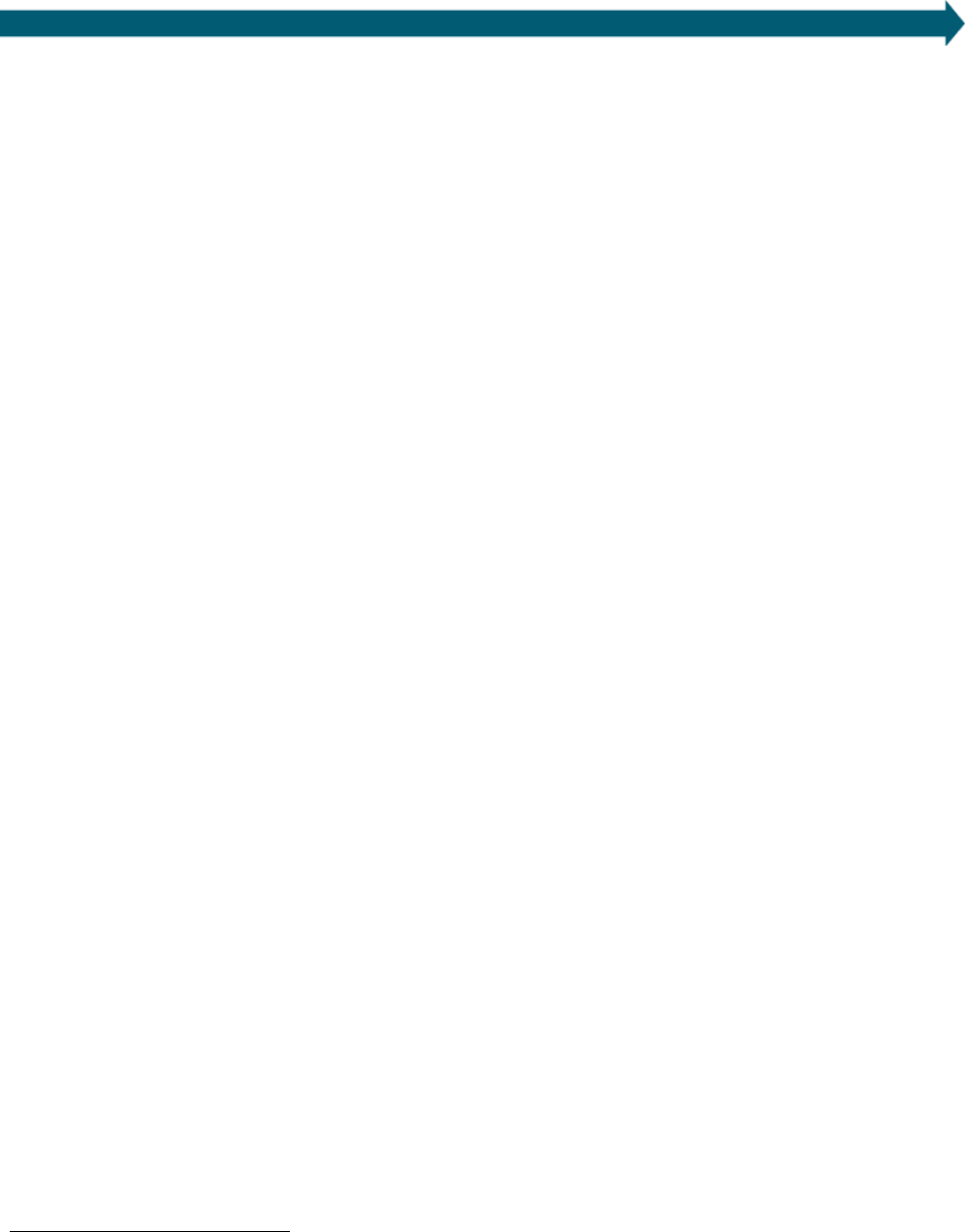
March 2022 www.seeaction.energy.gov 19
relationships varies somewhat, in all cases higher credit scores are associated with lower chances of delinquency
and charge-off, and in all cases the relationships are statistically significant at conventional levels of significance.
3.2.3.2 Income regression results
Across all portfolios combined, relative to loans in the 0-60% AMI band, the chance of charge-off decreases for
loans in the three highest income bands (80-100% AMI, 100-120% AMI, > 120% AMI). These differences are
statistically significant. Holding all else equal, households in the > 120% AMI band have a charge-off rate 1.98
percentage points lower than those in the < 60% AMI band. When examining delinquency, loans in the two highest
income bands (100-120% AMI, > 120% AMI) show statistically significantly lower rates of delinquency than loans in
the lowest income band. Both the high-income bins show a rate lower by 0.50 percentage points relative to the
rate in the <60% AMI band (see Table A-2).
In single-portfolio regressions, the impacts of income on charge-off were often, but not always, statistically
significant. The impact of income on delinquency were almost never statistically significant. This difference
between pooled and single-portfolio results suggests that the scale of the study – in these regressions (which do
not include Keystone HELP), about 25,000 loans – is important to demonstrate a relationship between income and
loan performance, especially in the case of delinquency. When pooling the programs, the relationships emerge;
however, single portfolios do not have adequate sample size to clearly demonstrate them. This stands in contrast
to credit score, where associations with both delinquency and charge-off are clear and large even in single-
portfolio regressions.
One possible explanation for the relatively weak relationship between income and loan performance (also shown
in Figure 12) is that the income variable is based on the median income of the census tract, rather than the income
of the household itself. Census tract income is a blunt signal of actual household-level income. However, the data
do not suggest that use of census tract incomes, rather than household incomes, is consequential for these results.
For Michigan Saves – the one program with available household-level income data – the correlation between
household income and census tract median income is relatively weak (0.20). However, the relationships between
household income and charge-off/delinquency are not clearly different than those using census tract median
incomes. Regression analysis on the Michigan Saves data shows that household incomes – like census tract median
incomes – are associated with charge-offs, with a $10,000 increase in income decreasing the chance of charge-off
by 0.26 percentage points. Household income is not a statistically significant predictor of delinquency in the
Michigan Saves data. Both results are similar to the results of regression analysis on the Michigan Saves program
using census tract incomes.
3.2.3.3 Program regression results
The regression analysis generally confirms the differences in overall program delinquency and loss rates presented
in Section 3.2.1. Connecticut Smart-E has the lowest charge-off rates when controlling for the other factors
discussed in this section, and NYSERDA’s Smart Energy loans have the highest.
15
Smart-E and both NYSERDA
programs have the highest delinquency rates, while Keystone and Michigan Saves have the lowest. It is beyond the
scope of this study to consider program-specific features that might explain these differences in performance.
Overall, while some of these differences are statistically significant, they are relatively small in magnitude.
3.2.3.4 Other regression results
In addition to credit score and income, the regression analysis also estimates the impact of principal amount,
interest rate, and seasoning on loan performance. This section reviews only the results for regressions on the
combined portfolios.
15
This study observes higher chances of delinquency in NYSERDA’s on-bill loans relative to its Smart Energy loans, consistent with the findings
in Deason (2015) several years earlier in the program’s lifespan. However, Deason (2015) did not study charge-offs. The results in this report
show that NYSERDA’s on-bill loans are not charged off more often than its Smart Energy loans; in fact, controlling for other factors, they are
charged off less often, though the difference is not statistically significant.